Simplifying the Path to Modernize Systems and Scale Analytics
“..providing wide access to data and analytics has long gone neglected in government’s modernization efforts.”
- State CIO1
“…what we’re essentially doing is putting a new system into legacy services and business processes and we’re siloing the data, only on a more modern system.”
- State CIO1
Failing to consider data and analytics requirements when public sector organizations replace their legacy system with a modern system:
- Compromises the unique business functionality built into the applications.
- Doesn’t account for new data types and formats
- Necessitates adoption of advanced analytics solutions which can be time consuming
It’s important to align application and analytics modernization initiatives because as one State CIO commented1, “if we wait until after we have finished our modernization project to ask what we need the system to tell us or what the system could tell us, it’s a missed opportunity. You’re now trying to retrofit data collection or data models to a system that you’ve already implemented.”
This blog explores how organizations can align application and analytics modernization initiatives through the Progressive Modernization approach.
Challenges Of Scaling Analytics with Legacy Systems
Data and analytics are challenging with legacy systems for the following reasons:
- Limited access to newer forms of data and analytics models and tools
- Difficult to access real-time data
- Time-intensive analytics and reporting
- Increased risk of data quality issues
- Difficulty integrating with new systems
All these challenges not only hamper analytics but also affect staff and constituent experience. Limited ability to undertake newer forms of data and analytics, time-intensive reporting, and the inability to access real-time data leave legacy system users behind in contemporary data-driven markets. Legacy systems also prevent organizations from improving their analytics maturity level as these systems are not easy to integrate and scale with advanced analytics products and innovative data science experiments, often needing a complete overhaul to move from a data-driven to a data-native level of analytics maturity.
Additional Read:
Progressive Modernization: Creating a Construct for Legacy Modernization & Advanced Analytics
In our last blog, we discussed how Progressive Modernization leverages a managed services model to – a) generate savings which can fund transformation, and b) gain better understanding of legacy applications, their workflows, and the data that they process.
Progressive Modernization enables organizations to make the most judicial use of this knowledge by transforming systems through non-invasive strategies like:
- Refactoring or Re-engineering - Modifying legacy applications to work within today’s cloud architecture. This approach retains an application’s functionality, while updating the underlying source code to take advantage of modern cloud-native functions, the associated flexibility, and adaptability. Organizations can systematically refactor critical applications and processes to align with the cloud environment without replacing the containers and the framework, and without any downtime or disruptions.
- Re-hosting - Also referred to as "lift and shift,” the re-hosting approach to application modernization involves redeploying an application’s underlying resources from on-premises servers or data centers to the cloud. Re-hosting does not involve any changes to the application’s underlying codebase and is a low-cost method for migrating less strategic and non-volatile applications to the cloud.
Both approaches retain the rich business knowledge that an organization has built over decades and at the same time unlocks the data that is trapped in the systems. Here is a high-level description of how the re-engineering option accelerates modernization and helps free up data:
- Automated tools reverse engineer the application to understand its functionality, extract business rules, and identify data being processed by the application
- Forward engineering tools re-write the application logic in modern, cloud-enabled technologies, and create APIs to make it easier to access data stored by the application
- Automated test generators ensure that the output at each stage is accurate and works as intended
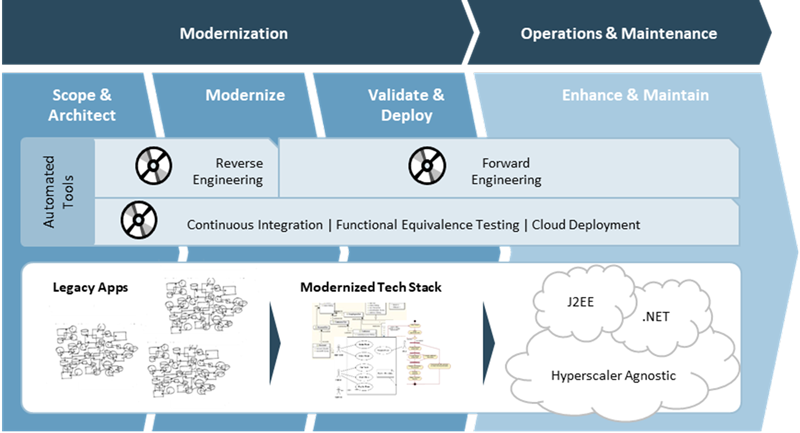
Scaling Analytics During and Post Modernization
Once the data is unlocked, Progressive Modernization leverages a three-phase approach – Evaluate, Optimize, Develop & Scale - to help organizations scale their analytics capabilities. The phases can run during or post modernization and include the following activities:
- Evaluate: Understand all the data sources organizations can potentially access, including the data that was trapped in legacy systems but is now available for analysis. Create a plan to transform the data estate, from a technology, people and process perspective.
- Optimize: Rationalize the existing analytics landscape and implement an agile, cloud-based, future-ready modern data backbone architecture for faster and more flexible access to large data volumes at speed from disparate sources.
- Develop and Scale: Extend the modern and resilient data backbone with next-gen data engineering, data management, governance and analytics capabilities. Bring disparate data together, apply business logic in governance and stewardship of the data, and make it available in curated formats for various downstream and advanced data analysis consumption use cases.
The three-phase approach enables organizations to evolve their analytics maturity, leverage data with varying efficiency, and generate different type of insights for strategic and tactical decision making:
- Data-driven organization: Data-driven organizations are at the procedural level of data analytics maturity, relying on legacy data architecture and solutions like a traditional data warehouse, data management, and dashboards and reporting. Data-driven organizations generally fall into the descriptive or diagnostic stage of analytics maturity, where data-driven insights guide well-informed and better strategic decisions.
- Data-native (or digital-native) Organization: Data-native organizations are at the proactive level of analytics maturity, utilizing the data insights to innovate and transform their core missions while reimagining the day-to-day operations. Relying on strategies and solutions like big data analytics platforms, digitizing data consumption via hybrid cloud strategy, and migration from legacy systems for modernization, data-native enterprises are at the predictive stage of the analytics maturity model. As a result, most of the analytics efforts focus on research-based advanced analytics platforms to improve agility and risk mitigation, transforming, and modernizing legacy platforms, enabling self-service analytics, unifying customer data for operational performance measurement analytics, and predictive modeling. Data-native enterprises are on the cusp of transformation, attempting to future-proof their position in the data economy.
- Organizations in the data economy: Organizations at the leading level of analytics maturity operate within the data economy, with analytics and artificial intelligence transforming the economy with data as the primary capital. At the prescriptive stage of analytics maturity, all stakeholders across agencies have equal access to data and analytics tools to draw insights for value addition and data-driven decision-making. As a result, the data economy revolutionizes how organizations tackle business priorities with AI-powered consumption, connected data, and a “digital” brain.[5]
Accelerating the digital modernization journey through next gen data ecosystems development brings the ability to support seamless extraction and migration of data from legacy sources, automating each stage of Data Lake and warehouse build, and enabling a loosely coupled architecture to cater to the plug and play model of data delivery and consumption use cases to support various advanced data science driven analytics projects -- like customer 360, fraud analytics, language model analysis, etc.
Wrapping Up
By scaling analytics capabilities, organizations are:
- 228% more likely to be agile
- 240% more likely to better serve constituents
As a result, more organizations are focusing on modernizing their legacy systems and incorporating analytics modernization to advance their analytics maturity level, progressing to becoming a part of the digital economy.
Modern solutions, like cloud-based modern data architecture and AI-powered data engineering, pipeline management, downstream analysis, and self-service analytics, can help fast-track this journey to the data economy at a significantly lower cost than rewriting or replacing legacy IT systems.
Related Reading: